Cohere and Qdrant Tutorial: Text Similarity Search
Mastering Qdrant: The Go-To Solution for Vector Similarity Applications
As a remarkable innovation in modern technology, Qdrant is a high-performance search engine and database tailored for vector similarity. Developed using Rust, Qdrant delivers fast, dependable performance even under rigorous workloads, making it a top choice for applications demanding speed and scalability.
Qdrant is more than just a databaseโit's a robust solution that can transform embeddings or neural network encoders into powerful, versatile applications. Whether you need to execute matching, searching, recommending, or other intricate operations on sizeable datasets, Qdrant is your one-stop solution.
One of Qdrant's distinguishing attributes is its comprehensive filtering support, which makes it ideally suited for faceted searches and semantic-based matching. When combined with its intuitive API, working with Qdrant has never been simpler.
Qdrant's convenience extends even further with Qdrant Cloud, a managed solution requiring minimal setup and maintenance. This enables seamless deployment and management of applications, reducing the burden on developers and administrators.
For an in-depth exploration don't miss our dedicated Qdrant AI tech page. Discover how Qdrant empowers developers to harness the potential of vector similarity in AI apps, leveling up your projects and setting them apart from the competition.
What will we do?
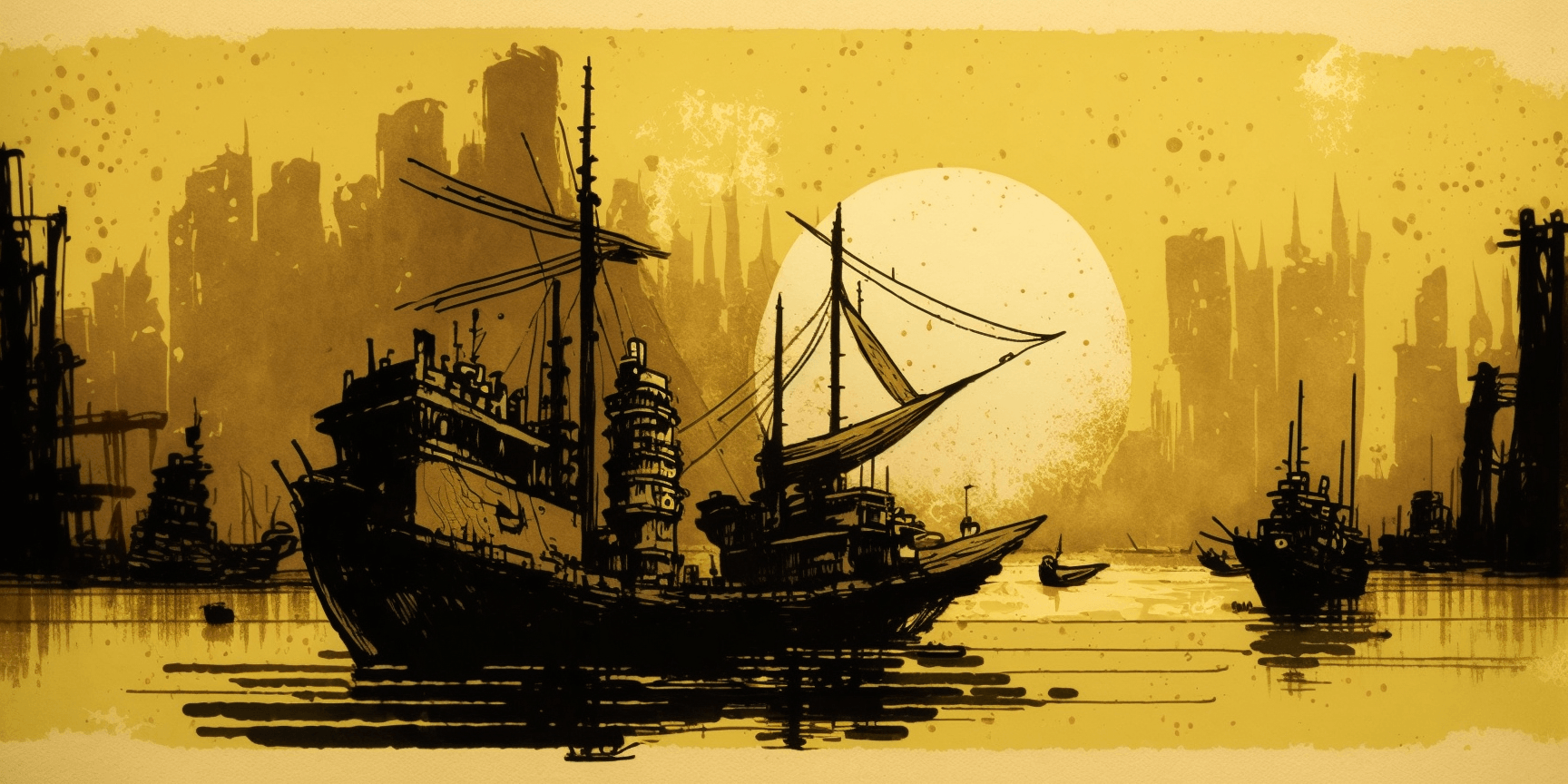
In this tutorial we will utilize Qdrant Vector Database to store Embeddings from Cohere's model and search using cosine similarity. We will use Cohere SDK to access the model. So, without any further ado, letโs jump in!
Prerequisites
I will use Qdrant Cloud to host my Database. And good to know, that Qdrant provides 1 GB of free forever memory. So go and use Qdrant Cloud. You can find out how to do it here.
Now let's create a new virtual environment inside project directory and install the required packages:
python3 -m venv venv
source venv/bin/activate
pip install cohere qdrant-client python-dotenv
Please create a project .py
file.
Data
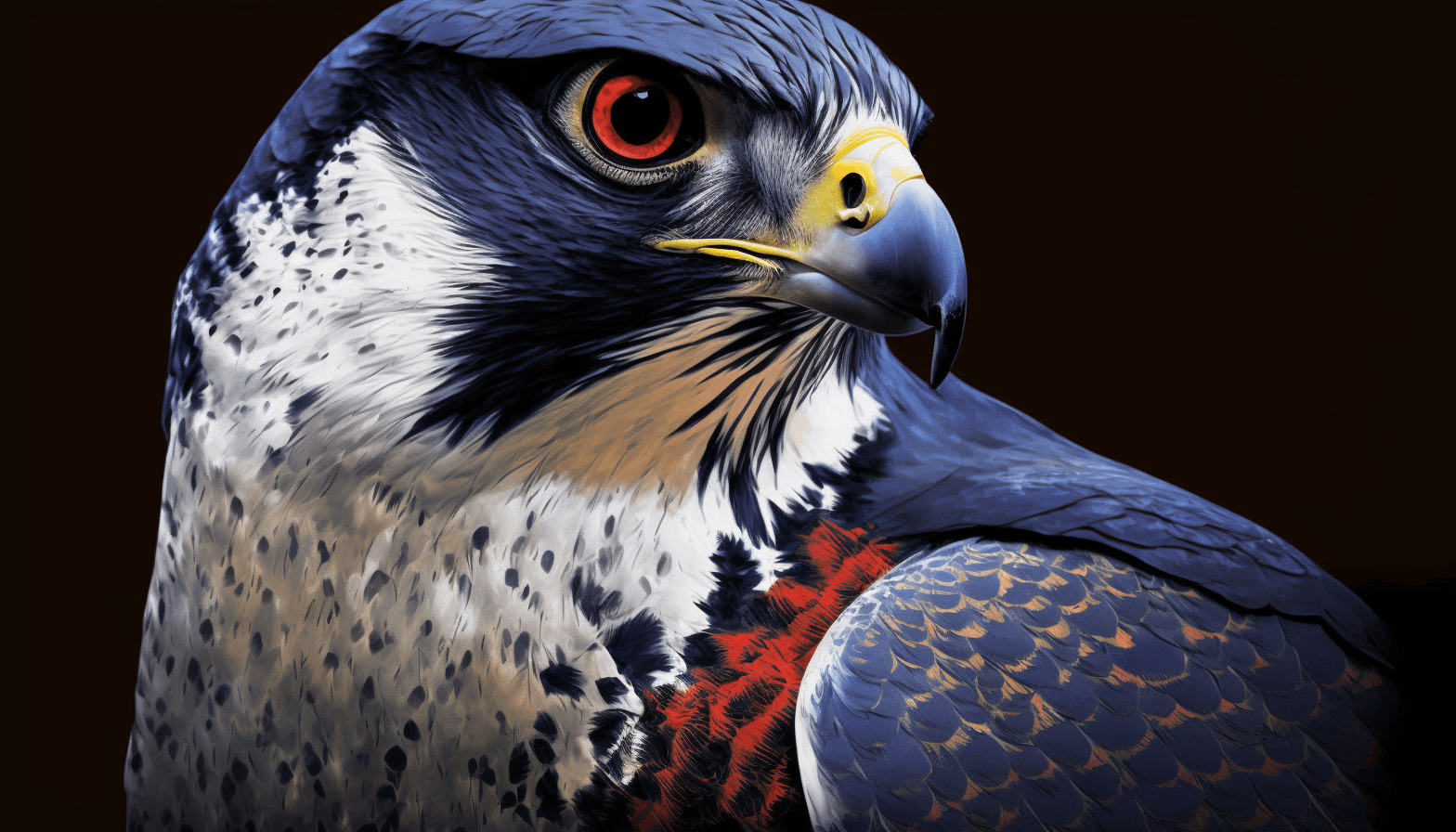
We will store our data in JSON format. Feel free to copy it:
[
{ "key": "Lion", "desc": "Majestic big cat with golden fur and a loud roar." },
{ "key": "Penguin", "desc": "Flightless bird with a tuxedo-like black and white coat." },
{ "key": "Gorilla", "desc": "Intelligent primate with muscular build and gentle nature." },
{ "key": "Elephant", "desc": "Large mammal with a long trunk and gray skin." },
{ "key": "Koala", "desc": "Cute and cuddly marsupial with fluffy ears and a big nose." },
{ "key": "Dolphin", "desc": "Playful marine mammal known for its intelligence and acrobatics." },
{
"key": "Orangutan",
"desc": "Shaggy-haired great ape found in the rainforests of Borneo and Sumatra."
},
{ "key": "Giraffe", "desc": "Tallest land animal with a long neck and spots on its fur." },
{
"key": "Hippopotamus",
"desc": "Large, semi-aquatic mammal with a wide mouth and stubby legs."
},
{ "key": "Kangaroo", "desc": "Marsupial with powerful hind legs and a long tail for balance." },
{ "key": "Crocodile", "desc": "Large reptile with sharp teeth and a tough, scaly hide." },
{
"key": "Chimpanzee",
"desc": "Closest relative to humans, known for its intelligence and tool use."
},
{ "key": "Tiger", "desc": "Striped big cat with incredible speed and agility." },
{ "key": "Zebra", "desc": "Striped mammal with a distinctive mane and tail." },
{ "key": "Ostrich", "desc": "Flightless bird with long legs and a big, fluffy tail." },
{ "key": "Rhino", "desc": "Large, thick-skinned mammal with a horn on its nose." },
{ "key": "Cheetah", "desc": "Fastest land animal with a spotted coat and sleek build." },
{
"key": "Polar Bear",
"desc": "Arctic bear with a thick white coat and webbed paws for swimming."
},
{ "key": "Peacock", "desc": "Colorful bird with a vibrant tail of feathers." },
{ "key": "Kangaroo", "desc": "Marsupial with powerful hind legs and a long tail for balance." },
{
"key": "Octopus",
"desc": "Intelligent sea creature with eight tentacles and the ability to change color."
},
{ "key": "Whale", "desc": "Enormous marine mammal with a blowhole on top of its head." },
{ "key": "Sloth", "desc": "Slow-moving mammal found in the rainforests of South America." },
{ "key": "Flamingo", "desc": "Tall, pink bird with long legs and a curved beak." }
]
Environment variables
Create .env
file and store your Cohere API key, Qdrant API key and Qdrant host there:
QDRANT_API_KEY=<qdrant-api-keu>
QDRANT_HOST=<qdrant-host>
COHERE_API_KEY=<cohere-api-key>
Importing libraries
import json
import os
import uuid
from typing import Dict, List
import cohere
from dotenv import load_dotenv
from qdrant_client import QdrantClient
from qdrant_client.http import models
Load Environment variables
load_dotenv()
QDRANT_API_KEY = os.getenv("QDRANT_API_KEY")
QDRANT_HOST = os.getenv("QDRANT_HOST")
COHERE_API_KEY = os.getenv("COHERE_API_KEY")
COHERE_SIZE_VECTOR = 4096 # Larger model
if not QDRANT_API_KEY:
raise ValueError("QDRANT_API_KEY is not set")
if not QDRANT_HOST:
raise ValueError("QDRANT_HOST is not set")
if not COHERE_API_KEY:
raise ValueError("COHERE_API_KEY is not set")
How to index data and search throught it later?
I will implement the SearchClient
class, which will be able to index and access our data. Class will contain all necessary functionalities, such as indexing and searching, but also data conversion to necessary formats.
class SearchClient:
def __init__(
self,
qdrabt_api_key: str = QDRANT_API_KEY,
qdrant_host: str = QDRANT_HOST,
cohere_api_key: str = COHERE_API_KEY,
collection_name: str = "animal",
):
self.qdrant_client = QdrantClient(host=qdrant_host, api_key=qdrabt_api_key)
self.collection_name = collection_name
self.qdrant_client.recreate_collection(
collection_name=self.collection_name,
vectors_config=models.VectorParams(
size=COHERE_SIZE_VECTOR, distance=models.Distance.COSINE
),
)
self.co_client = cohere.Client(api_key=cohere_api_key)
# Qdrant requires data in float format
def _float_vector(self, vector: List[float]):
return list(map(float, vector))
# Embedding using Cohere Embed model
def _embed(self, text: str):
return self.co_client.embed(texts=[text]).embeddings[0]
# Prepare Qdrant Points
def _qdrant_format(self, data: List[Dict[str, str]]):
points = [
models.PointStruct(
id=uuid.uuid4().hex,
payload={"key": point["key"], "desc": point["desc"]},
vector=self._float_vector(self._embed(point["desc"])),
)
for point in data
]
return points
# Index data
def index(self, data: List[Dict[str, str]]):
"""
data: list of dict with keys: "key" and "desc"
"""
points = self._qdrant_format(data)
result = self.qdrant_client.upsert(
collection_name=self.collection_name, points=points
)
return result
# Search using text query
def search(self, query_text: str, limit: int = 3):
query_vector = self._embed(query_text)
return self.qdrant_client.search(
collection_name=self.collection_name,
query_vector=self._float_vector(query_vector),
limit=limit,
)
Let's use our code!
Let's try to read data from the data.json
file, process and index it. Then we can try to search and get top 3 results from our Database!
if __name__ == "__main__":
client = SearchClient()
# import data from data.json file
with open("data.json", "r") as f:
data = json.load(f)
index_result = client.index(data)
print(index_result)
print("====")
search_result = client.search(
"Tallest animal in the world, quite long neck.",
)
print(search_result)
RESULTS!
operation_id=0 status=<UpdateStatus.COMPLETED: 'completed'>
===
[ScoredPoint(id='d17eb61c-8764-4bdb-bb26-ac66c3ffa220', version=0, score=0.8677041, payload={'desc': 'Tallest land animal with a long neck and spots on its fur.', 'key': 'Giraffe'}, vector=None), ScoredPoint(id='4934a842-8c55-42bc-938f-a839be2505de', version=0, score=0.71296465, payload={'desc': 'Large, semi-aquatic mammal with a wide mouth and stubby legs.', 'key': 'Hippopotamus'}, vector=None), ScoredPoint(id='05d7e73c-a8bf-44f9-a8b4-af82e06719d0', version=0, score=0.69240415, payload={'desc': 'Large, thick-skinned mammal with a horn on its nose.', 'key': 'Rhino'}, vector=None)]
As you can see in 1st row: index operation went well. We got 3 results, as we defined. The 1st one is (as expected) a Giraffe. We also got Hippopotamus and Rhino. They are also huge, but I think Giraffe is the tallest ๐.
The Next Steps: Harnessing Qdrant and Cohere for Your AI Applications
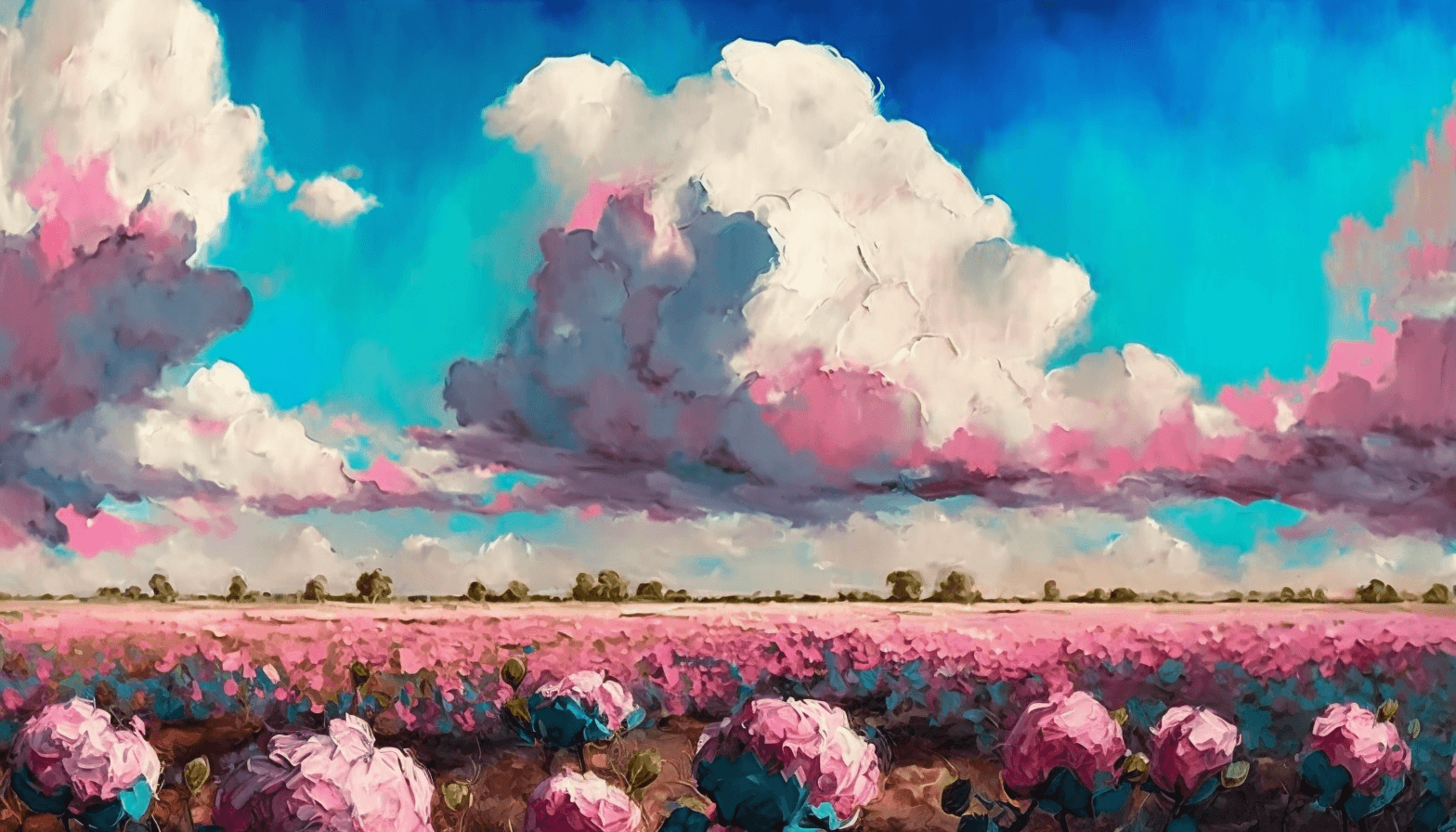
So, right now you've got the basics of Qdrant under your belt. What's next on your AI journey? It's time to put your newfound skills to the test. Consider building an API that allows your application to index data, add new records, and search. FastAPI is a fantastic tool for this task, offering a high-performance, easy-to-use framework for building APIs.
But don't stop there. If you're ready to take your skills to the next level, why not use them to build an AI application during upcoming AI Hackathons. Those events bring together a community of innovators and creators, all eager to shape the future with AI. It's a chance to learn, grow, and maybe even create something groundbreaking. During a couple of days, you align with people from all around the world and create a solution to an existing problem!
Don't forget to check out our other events too. We're always hosting exciting opportunities for our community to learn, innovate, and push the boundaries of what's possible with AI.
Thank you for your time! - Jakub Misiลo @newnative